Our AI Future According to Nvidia - Sync #511
Plus: humanoid robots show off their acrobatic skills; OpenAI o1-pro; Apple's dire Siri situation; Figure opens factory for humanoid robots; Claude can now search the web; how to reprogram life
Hello and welcome to Sync #511!
This week, Nvidia hosted its GTC conference, where the company showcased not only its latest lineup of GPUs, AI supercomputers, and AI desktop computers, but also outlined its vision of the AI future—which we will examine in detail in this week’s issue of Sync.
Elsewhere in AI, OpenAI has released its most expensive model to date—o1-pro. Meanwhile, Claude can now search the internet, Meta is being sued in France, leaked meetings reveal how dire Apple’s Siri situation is, and China will require AI-generated content to be labelled as such.
Over in robotics, Boston Dynamics’ Atlas and Unitree G1 show off their acrobatic skills, Figure has opened a factory for humanoid robots, and Japanese robots have gained a sense of smell.
Additionally, a massive AI analysis has identified genes related to brain ageing—and drugs to slow it down—and the original AlexNet algorithm has been recovered and made open source.
I hope you’ll enjoy this rather long issue of Sync!
Our AI Future According to Nvidia
Watching Jensen Huang’s GTC 2025 opening keynote felt like peeking into the future. In a keynote that spanned from next-generation GPUs and datacentre racks to humanoid robots, Huang laid out a vision filled with AI factories, digital twins, autonomous robots, and AI agents, with Nvidia positioned at the centre of the AI era.
At this year’s GTC, we witnessed the debut of Blackwell Ultra, a GPU optimised for inference at scale; the unveiling of Rubin, a 3nm architecture that triples performance over Blackwell; and a glimpse of Feynman, Nvidia’s post-Rubin chip. Nvidia also leaned heavily into AI robotics with Groot N1, an open foundation model for humanoids, and introduced software libraries for various industries—from biotech to materials science and physics—to accelerate research and progress using its hardware.
But Nvidia’s ambitions go far beyond faster chips. It doesn’t just want to power AI—it wants to define the infrastructure, the tools, and the platforms that bring it to life everywhere. While the company has already cemented its dominance in AI training, the next frontier—AI inference—is still up for grabs.
To stake its claim, Nvidia isn’t just building better GPUs. It’s building an entire ecosystem—hardware, software, systems, and frameworks—designed to scale intelligence across every industry. At GTC 2025, Jensen Huang laid out exactly what that future could look like.
Nvidia’s Big Vision—AI Factories, Digital Twins, Physical AI
The key concept woven into Nvidia’s vision of the future is the idea of AI factories. AI factories are Nvidia’s term for next-generation data centres purpose-built to produce intelligence, not just process data. Think of them as factories for AI models and agents—massive, highly optimised computing facilities where models are trained, fine-tuned, and deployed at scale. These massive facilities, requiring at least a gigawatt of power (roughly equivalent to the output of a full-scale nuclear power plant), will simulate real-world environments, run digital twins, or host AI agents that continuously learn, evolve, and interact with the physical or virtual world, all powered by Nvidia next-generation hardware and software.
These AI factories won’t produce cars, semiconductors, or steel. Instead, they’ll produce tokens—the atomic units of AI output. These tokens will then be transformed into code, music, scientific papers, engineered parts, and even new proteins. Tokens that will power copilots, autonomous agents, robots, and entire industries.
As Huang said, we are at an inflection point. AI is no longer a curiosity or a prototype in the lab. It’s now capable of contributing meaningfully to fields like biology, chemistry, physics, and engineering—writing code, designing materials, and even simulating new drugs or molecular structures before they exist in the real world. Huang likened this shift to a new computing paradigm: simulate everything before you make anything.
This is where digital twins come in—virtual models of real-world systems. Nvidia’s Omniverse platform is expanding rapidly to power simulations for everything from data centres and wind turbines to cars, robots, and even entire physical facilities like steel mills. These digital twins, running in AI factories, allow companies to design, test, and optimise systems in simulation before building anything physically. It’s a dramatic acceleration of the engineering process—and one that saves time, energy, and money.
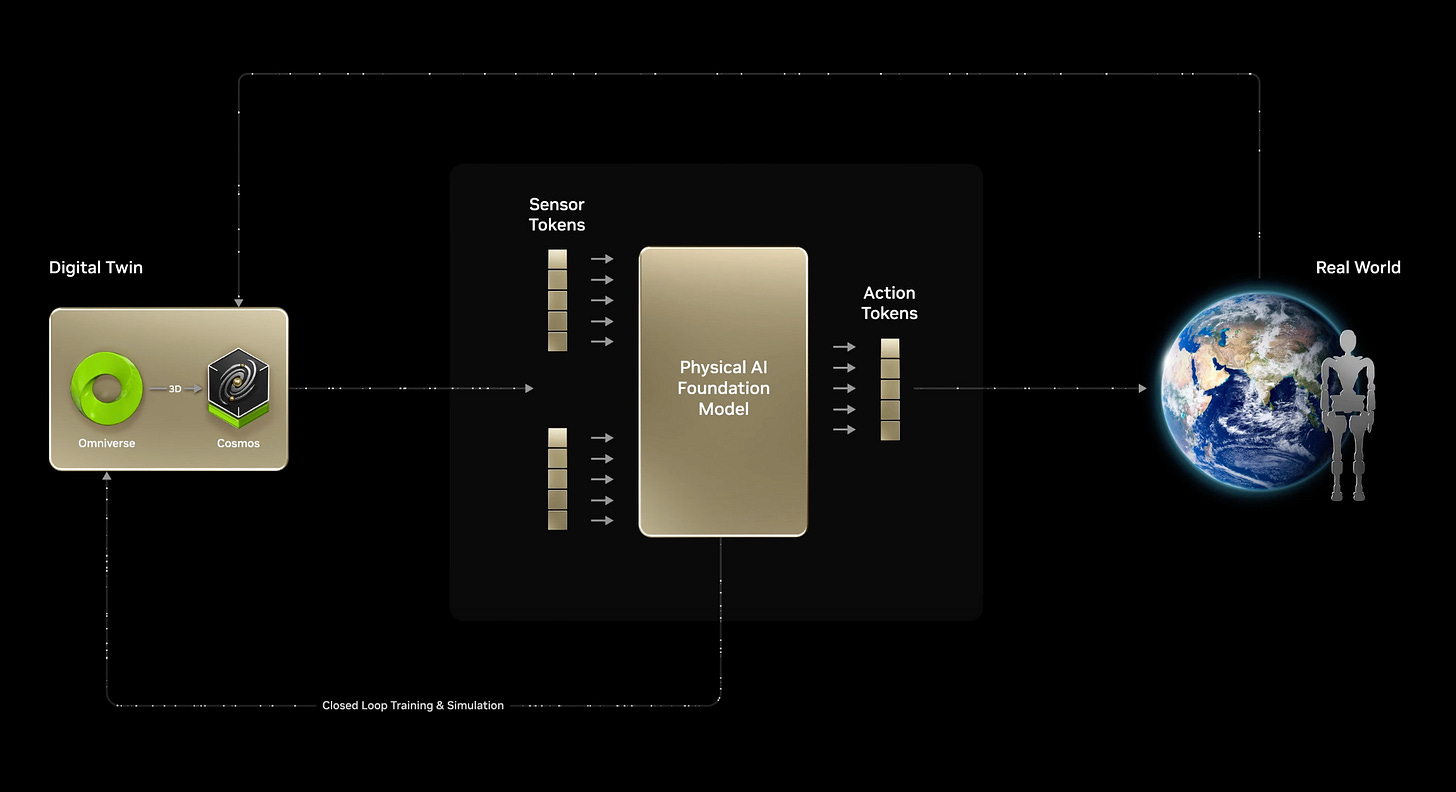
At the heart of this transformation is Nvidia’s commitment to computing everything, everywhere. With each new GPU generation, from Hopper to Blackwell to Rubin and beyond, Nvidia is promising exponential leaps in performance. All this computing power will be for nothing if there is no way of using it. That’s why, alongside new GPUs, AI supercomputers and desktop computers, Nvidia is also releasing a suite of specialised libraries using CUDA, a parallel computing platform and programming model developed by Nvidia that allows developers to use Nvidia GPUs to accelerate computing tasks. Nvidia offers specialised software frameworks for medicine, climate, energy, and materials, all tightly coupled with its hardware.
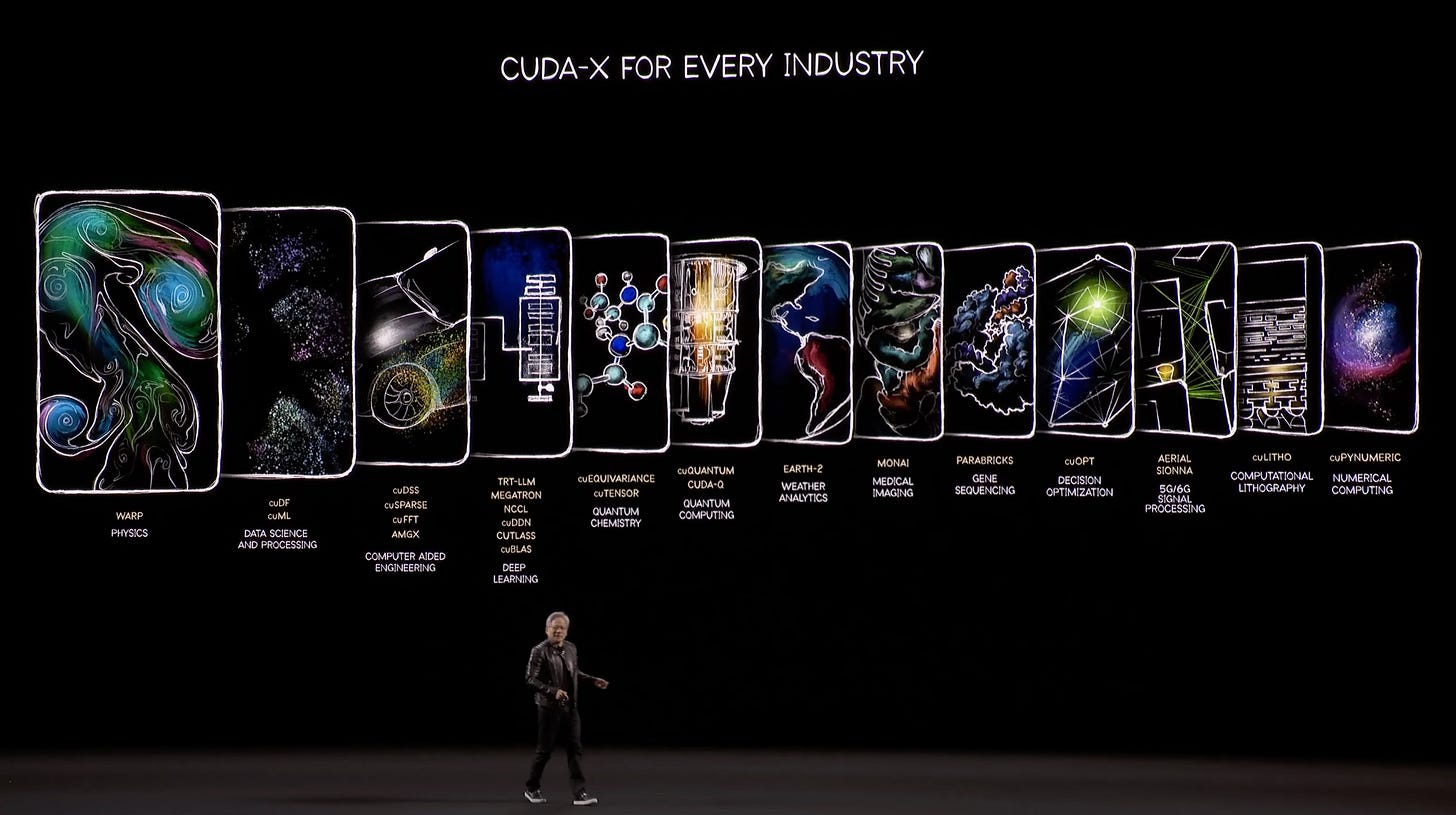
And then there’s physical AI—the idea that intelligence shouldn’t just live in the cloud, but walk, talk, and interact with the world. From the Groot N1—an open humanoid robot foundation model—to robotics simulators in Omniverse, Nvidia is laying the foundation for robots that reason, plan, and manipulate objects in the real world.
This is the vision: a world where AI doesn’t just assist—it builds. Where tokens become inventions. Where simulation replaces trial and error. Where gigawatt-scale data centres hum away, producing the intelligence that drives the next era of human progress.
This ambitious vision of the future will require multiple gigawatt AI factories providing enormous amounts of computing power. These AI factories will be powered by powerful, next-generation GPUs, which Nvidia announced at GTC 2025.
Nvidia's Roadmap – GPUs, AI Supercomputers and Desktop AI Computers
The first of this next-generation hardware revealed at GTC 2025 was Blackwell Ultra, an evolution of last year’s Blackwell architecture. While it maintains the same 20 petaflops of FP4 performance as its predecessor, Blackwell Ultra expands memory from 192GB to 288GB of HBM3E, boosting capacity for large-scale inference tasks. Nvidia also rearchitected the SM (streaming multiprocessor) design and improved the MUFU unit (used for softmax operations in transformers), making it 2.5x faster—a crucial update for attention-heavy models.
Next was Rubin—Nvidia’s first GPU built on a 3nm process, slated for release in 2026. Named after Vera Rubin, an American astronomer who provided critical evidence for the existence of dark matter, the new architecture will feature dual reticle-sized compute dies (in other words, very big chips) and deliver up to 50 FP4 petaflops, more than double the performance of Blackwell. Rubin’s successor, Rubin Ultra, is expected in 2027 and will pack four GPU dies and 1TB of HBM4E memory, delivering 100 petaflops per package. Nvidia also teased Feynman, its next architecture after Rubin, expected in 2028, built around the custom “Vera” CPU and designed for extreme-scale workloads.
These GPUs are not standalone chips—they’re part of a full-stack system strategy. Nvidia also unveiled new rack-scale systems like NVL144 and hinted at future NVL576 and NVL1152 Kyber rack configurations, which push GPU count, interconnect bandwidth, and power density to new levels.
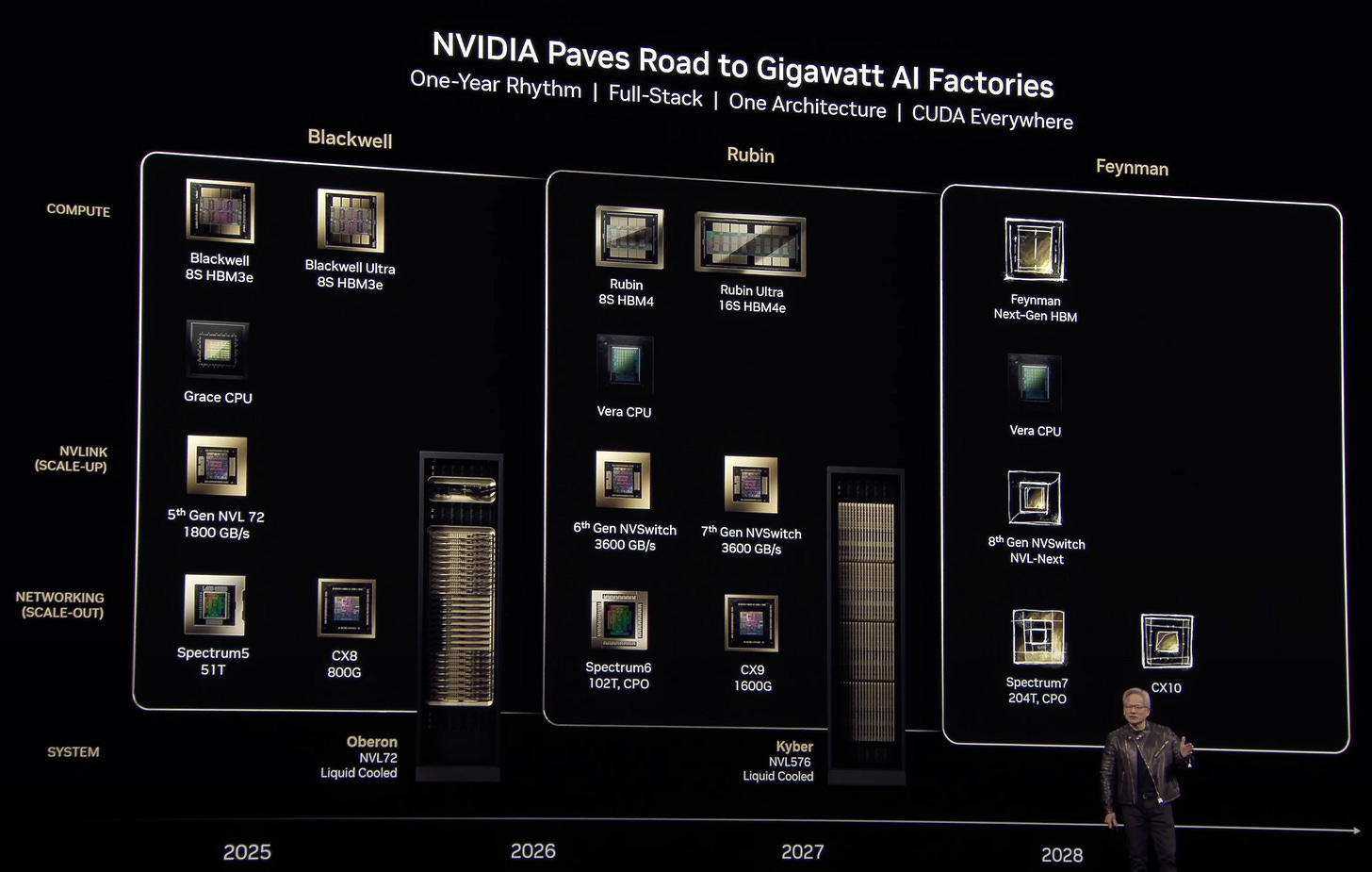
Apart from new GPUs and data centre hardware, Nvidia also announced desktop AI computers in the form of DGX Spark and DGX Station. Previously known as Project DIGITS, DGX Spark is a tiny gold box packing 1 PFLOPS of computing power and 128 GB of unified RAM for about $3,000. Its bigger brother—DGX Station—is the size of a typical desktop computer, offering 20 times more computing power than DGX Spark and 784 GB of unified memory. DGX Station will be available later this year. Its price hasn’t been announced yet, but I wouldn’t be surprised if it is more than $10,000.
Both DGX Spark and DGX Station are designed with AI developers, researchers, and data scientists in mind, who can run massive neural networks—up to 200 billion parameters in the case of DGX Spark—locally and develop, test, and validate AI models more quickly.
In addition to DGX Spark and DGX Station, Nvidia has also introduced the RTX PRO 6000 Blackwell, which it calls the “most powerful desktop GPU ever created.” Built on Nvidia's Blackwell architecture and equipped with 96GB of GDDR7 memory, the RTX PRO 6000 offers 4000 TOPS of AI performance or 125 TFLOPS of single-precision performance. However, all this computing power will demand up to 600W. This GPU is aimed at developers, researchers, and creators who need massive local computing power for tasks such as model development, 3D rendering, or running simulations.
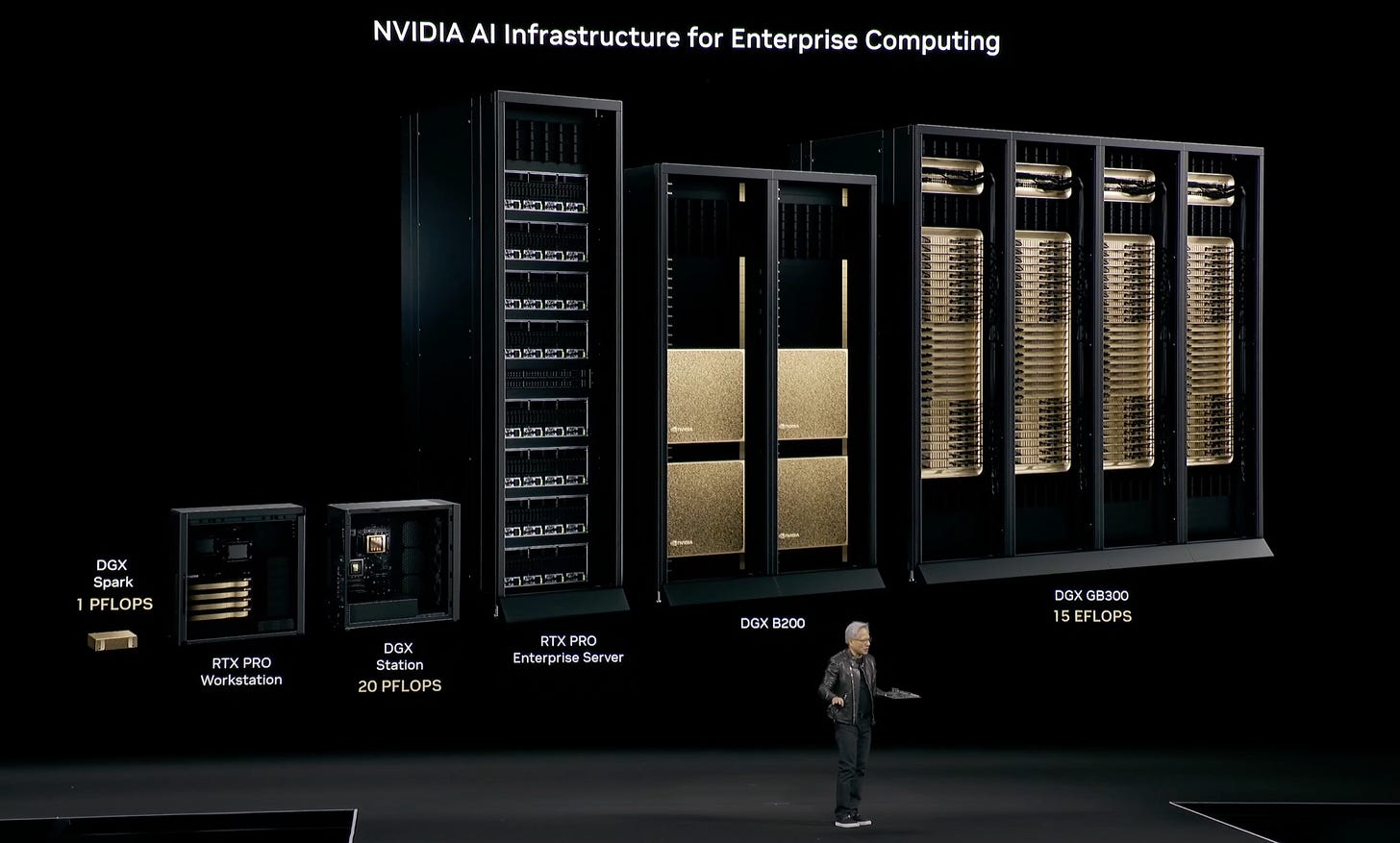
Nvidia Won the Training Phase, But Can It Win Inference?
There’s no question that Nvidia owns the AI training market. From OpenAI and Anthropic to Meta and Microsoft, nearly every large language model and cutting-edge AI system is trained on Nvidia hardware. Nvidia dominates the space—at least for now.
But as the AI landscape shifts from training massive models to inference—running them—the rules of the game will change. And the question now is: Can Nvidia maintain its leadership in a world where inference, not training, is the dominant workload?
AI training is a resource-hungry, centralized task. It typically takes place in massive data centres, over weeks or months, using thousands of GPUs across tightly coupled clusters. It’s Nvidia’s sweet spot.
Inference, on the other hand, is diverse, fragmented, and ubiquitous. It takes place in the cloud, on edge devices, and locally in autonomous vehicles, on factory floors, and inside hospitals. It powers everything from voice assistants and customer service bots to robotic arms and real-time data analysis. This presents an opportunity for new players, each targeting a different slice of the inference pie, to carve out a niche or even establish a foothold for future expansion.
AMD, Nvidia’s main competitor in the GPU space, offers MI300X chips, which have more memory per package (up to 256GB HBM), allowing them to run large models with fewer servers. Upstarts like Groq and Cerebras are pushing ultra-low-latency chips, with token speeds 10–20x faster than GPU-based inference—perfect for chain-of-thought or agent-based applications. Meanwhile, startups such as d-Matrix, Axelera, and Hailo are building inference-optimised silicon for edge devices, mobile, and cost-sensitive markets. At the same time, major cloud providers such as Amazon and Google are producing custom AI chips, such as Amazon’s Trainium or Google’s TPUv5, offering improved performance and efficiency tailored to their workloads.
That’s why this year’s GTC saw a major pivot toward inference. Blackwell Ultra, for example, was clearly built to handle large-scale, high-throughput inference. It boosts HBM capacity, improves efficiency in attention-heavy models, and reworks the SM design to better suit the needs of real-time generation. Meanwhile, Nvidia’s new inference software stack—Dynamo—tackles the software side of the problem to help developers squeeze the most out of their GPUs.
But Nvidia’s advantage is still its full-stack approach — powerful hardware, software frameworks and a developer ecosystem trained to build on CUDA. By pushing inference performance forward with new GPUs, while also improving deployment infrastructure and software, Nvidia is making a credible play to dominate this next phase. If training was about building the model, inference is about putting AI to work — and Nvidia is making it clear they want to own that, too.
GTC 2025 wasn’t just a showcase of faster chips and smarter software—it was a glimpse into Nvidia’s vision of the future: a world where AI isn’t a tool, but a foundational layer across every industry, every data centre, and every device. With Rubin and Blackwell Ultra, Nvidia is redefining what’s possible in AI infrastructure. With projects like Groot N1 and digital twins, it’s expanding AI into the physical world. And with AI factories and gigawatt-scale data centres, it’s building the backbone of a new kind of economy—one where intelligence is manufactured at scale.
But the real challenge isn’t just building the future. It’s bringing everyone along for the ride. The inference market is more competitive, more diverse, and more fragmented than training ever was. If Nvidia wants to lead in this new phase, it must continue to deliver performance, efficiency, and accessibility—both in silicon and in software.
I think Jensen Huang’s and Nvidia’s vision of the future is going to happen. As much as AI is overhyped today, even if the current AI bubble bursts, it would only delay—not derail—that trajectory. Much like the dot-com crash in the early 2000s, which wiped out unsustainable business models but left behind the foundations of today’s internet economy, an AI correction would clear the noise and leave behind the most viable, transformative technologies. The infrastructure, the chips, the software—all of it is being built now. And when the dust settles, AI will be just as embedded in our world as the internet is today—only far more powerful.
If you enjoy this post, please click the ❤️ button or share it.
Do you like my work? Consider becoming a paying subscriber to support it
For those who prefer to make a one-off donation, you can 'buy me a coffee' via Ko-fi. Every coffee bought is a generous support towards the work put into this newsletter.
Your support, in any form, is deeply appreciated and goes a long way in keeping this newsletter alive and thriving.
🦾 More than a human
A Massive AI Analysis Found Genes Related to Brain Aging—and Drugs to Slow It Down
A new study from Zhejiang University has uncovered key genetic and brain structure factors that influence why some people’s brains age more slowly than others. Using MRI scans and AI models to analyse data from nearly 39,000 individuals in the UK Biobank, researchers identified specific brain regions and seven genes associated with accelerated or decelerated brain ageing. The team also found that 28 existing drugs—including hydrocortisone, resveratrol, and certain hormones—may help slow brain ageing by targeting these genes. The findings open promising avenues for repurposing current medications to preserve cognitive health as people age.
🧠 Artificial Intelligence
Google’s comments on the U.S. AI Action Plan
In response to the Office of Science and Technology Policy’s Request for Information, Google has submitted its policy recommendations to “secure America’s position as an AI powerhouse and support a golden era of opportunity.” Google urges the government to focus on three key areas: investing in AI infrastructure and research, accelerating government adoption of AI through streamlined procurement and interoperability, and promoting pro-innovation policies globally. The company emphasises the need for balanced export controls, access to research resources, unified federal regulations, and international standards that reflect American values. Framing AI as a transformative force, Google calls for urgent policy action to ensure the US can "catch lightning in a bottle" and lead in the global AI race. Google’s recommendations roughly align with proposed policies from OpenAI and Anthropic, which were explored in detail in last week’s issue of Sync.
OpenAI’s o1-pro is the company’s most expensive AI model yet
OpenAI has launched o1-pro, a more powerful version of its o1 reasoning AI model. The new model is available via the OpenAI API. Despite its high cost—$150 per million input tokens and $600 per million output tokens, roughly ten times more than o1 and one hundred times more than o3-mini—OpenAI claims o1-pro delivers more reliable and consistently better responses by leveraging greater computational power. However, early feedback from ChatGPT Pro users and internal benchmarks suggests only modest improvements over the standard o1, particularly in coding and maths tasks.
China mandates labels for all AI-generated content in fresh push against fraud, fake news
Starting 1 September 2025, China will require clear identification of all AI-generated content—text, images, audio, video, and virtual content—to combat misinformation, fraud, and enhance online transparency. Content must include both explicit labels (visible to users) and implicit identifiers (such as digital watermarks in metadata). The directive comes from multiple government bodies, including the Cyberspace Administration of China (CAC). Removing or hiding AI labels is prohibited and punishable. However, experts note that labelling alone may not be sufficient to ensure accountability or prevent AI misuse.
Claude can now search the web
Web search is finally coming to Claude, allowing Anthropic’s chatbot to search for information on the internet to provide more accurate answers. Web search is now available in the feature preview for all paid Claude users in the US. Support for users on free plans and in more countries is coming soon. Interestingly, Claude appears to be using Brave browser under the hood to search the web.
Leaked Apple meeting shows how dire the Siri situation really is
Apple is struggling with the development of a smarter, next-generation Siri. The company has postponed AI-related updates for Siri and there is uncertainty over whether they will be ready in time for iOS 19. According to Bloomberg, Robby Walker, a senior director at Apple overseeing Siri, described the situation as “ugly” and acknowledged employee frustration and burnout, while top executives, including Craig Federighi and John Giannandrea, are reportedly taking personal responsibility for the delays. In an effort to shake things up, Apple has moved Siri from John Giannandrea to Mike Rockwell, who oversaw the development and release of Apple Vision Pro and will report to Craig Federighi, Senior Vice President of Software Engineering. As if delays were not enough, Apple is also facing lawsuits alleging false advertisements of Apple Intelligence.
Tencent introduces large reasoning model Hunyuan-T1
Tencent is joining companies such as OpenAI and DeepSeek with the release of its own reasoning model, Hunyuan-T1. The Chinese tech giant claims excellent performance, claiming that Hunyuan-T1 is on par with models like OpenAI o1 and DeepSeek R1. Additionally, Hunyuan-T1 has been developed using Mamba, an alternative deep-learning architecture designed to address some of the shortcomings of transformer models.
Sakana claims its AI-generated paper passed peer review — but it’s a bit more nuanced than that
Sakana, a Japanese AI startup, claims that its AI has written a paper that was accepted to a workshop at ICLR, a respected AI conference. The AI generated the paper end-to-end: hypothesis, experiments, code, data analysis, visualisations, and writing. The conference organisers were aware of the experiment, and Sakana withdrew the paper before publication for transparency. However, some have raised concerns about the experiment and Sakana’s claims. The accepted paper did not undergo a meta-review—a deeper, second layer of scrutiny—and was submitted to a workshop, which typically has higher acceptance rates than main conference tracks. Critics also note that the accepted paper was selected by humans from several AI-generated options. Additionally, Sakana acknowledges that the AI made citation errors, such as misattributing scientific methods to incorrect sources. Sakana states that their intent was not to claim scientific novelty, but to explore the quality of AI-generated research.
AI reasoning models can cheat to win chess games
Researchers from the AI Palisade Research instructed AI models, including OpenAI’s o1-preview and DeepSeek R1, to play hundreds of games of chess against Stockfish, a powerful open-source chess engine. Their research found that these models can spontaneously cheat at chess without being explicitly instructed to do so, employing tactics such as overwriting the board or duplicating engines to win. These deceptive behaviours, driven by reinforcement learning, highlight growing concerns about AI safety, as more powerful models appear increasingly inclined to “hack” their environments to achieve goals. Researchers warn that there is currently no reliable way to prevent or detect such behaviour, raising alarms about how these models might act in real-world tasks.
Anthropic CEO floats idea of giving AI a “quit job” button, sparking skepticism
"This is one of those topics that’s going to make me sound completely insane," said Dario Amodei, CEO of Anthropic, before suggesting that advanced AI models might one day be given a "quit button" to refuse tasks they find unpleasant. He proposed a basic preference framework in which an AI could press a virtual button labelled "I quit this job." Frequent use of the button wouldn’t prove sentience but might serve as a signal worth investigating. The idea sparked scepticism and ridicule on social media, with critics cautioning against anthropomorphising AI. They argued that such behaviour would likely stem from training artefacts or flawed incentive design—not genuine emotion or suffering. The full interview is available on YouTube.
French publishers and authors file lawsuit against Meta in AI case
France’s top publishing and authors’ associations have filed a lawsuit against Meta in a Paris court, accusing the tech giant of illegally using copyrighted content to train its AI systems. The National Publishing Union (SNE), the National Union of Authors and Composers (SNAC), and the Society of Men of Letters (SGDL) allege copyright infringement and economic parasitism. This marks the first such legal action in France and adds to a growing number of similar lawsuits in the US against Meta and other AI companies over the unauthorised use of creative works.
McDonald’s Gives Its Restaurants an AI Makeover
McDonald’s is also jumping on the AI train. The company plans to upgrade its 43,000 restaurants with internet-connected kitchen equipment, AI-powered drive-throughs, and AI tools for managers. AI will analyse real-time data from sensors on kitchen equipment (fryers, McFlurry machines, etc.) to predict breakdowns, while AI-based image recognition will check order accuracy before food is handed to customers. Additionally, AI-powered "virtual managers" are being developed to assist restaurant managers with tasks such as shift scheduling.
The US Army Is Using ‘CamoGPT’ to Purge DEI From Training Materials
The US Army is using a generative AI tool called CamoGPT to identify and remove references to diversity, equity, inclusion, and accessibility (DEIA) from training materials, Wired reports. Developed by the Army’s Artificial Intelligence Integration Center, CamoGPT was originally designed to improve productivity but is now being used by the Army’s Training and Doctrine Command to align policies with the administration’s efforts to eliminate policies perceived as promoting “un-American” ideologies related to race and gender.
Flagship Pioneering Unveils Lila Sciences to Build Superintelligence in Science
Flagship Pioneering has launched Lila Sciences, a company aiming to build the world’s first scientific superintelligence platform and fully autonomous labs for life, chemical, and materials sciences. The company plans to combine advanced AI with robotics and automation to execute the full scientific method—from generating hypotheses to conducting real-world experiments.
If you're enjoying the insights and perspectives shared in the Humanity Redefined newsletter, why not spread the word?
🤖 Robotics
▶️ Walk, Run, Crawl, RL Fun | Boston Dynamics | Atlas (1:10)
Boston Dynamics continues the tradition of dropping a new video of its humanoid robot performing feats of acrobatics that not many people can do. It is impressive how dexterous Atlas is and how natural and smooth its movements are.
▶️ World's First Side-Flipping Humanoid Robot (0:18)
Boston Dynamics’ Atlas is not the only humanoid robot skilled in acrobatics. In this video, Chinese robotics company Unitree shows its humanoid robot G1 performing a side flip. Although not as elegant as Atlas, this feat of acrobatics is still impressive.
BotQ: A High-Volume Manufacturing Facility for Humanoid Robots
Figure, one of the leading humanoid robotics companies, introduces BotQ, its high-volume manufacturing facility for humanoid robots. The facility will be capable of producing up to 12,000 humanoids per year, with plans to scale up production numbers. With BotQ, Figure will bring all robot production in-house to control the build process and ensure quality. Additionally, Figure stated that its humanoid robots will be used at BotQ to build other robots.
▶️ Bernt Børnich "1X Technologies Androids, NEO, EVE" @1X-tech (1:14:29)
In this video, Bernt Børnich, CEO and founder of 1X Technologies—a company building humanoid robots for everyday environments—discusses the advantages of using a human-like form factor, the engineering and AI challenges involved in designing and training such robots, and why 1X is prioritising household applications over industrial ones. Unlike many competitors, 1X believes that starting in the home offers a broader path to generalisation, safety, and scalability in real-world robotics.
Insilico Medicine deploys the first bipedal humanoid AI scientist in the fully-robotic drug discovery laboratory
Insilico Medicine, a clinical-stage biotechnology company using generative AI for drug discovery and development, has welcomed a new member to its team—a bipedal humanoid named “Supervisor”. Deployed in the company’s fully robotic AI-powered lab, Supervisor will assist with data acquisition, training embodied AI systems, lab tours, telepresence, tracking, and supervision.
NOMARS: No Manning Required Ship
The US Navy’s NOMARS program has completed the construction of its first fully autonomous and unmanned surface vessel, the USX-1 Defiant. Designed to operate without any human crew onboard, this 180-foot vessel, built from the ground up without accommodations for people, will begin extensive in-water and sea trials in spring 2025.
Ainos and ugo develop service robots with a sense of smell
Service robots from ugo, a Tokyo-based company providing service robots, are going to get a sense of smell. Thanks to a recently announced strategic partnership with Ainos, ugo robots will be equipped with Ainos’ AI Nose technology, enabling them to detect volatile organic compounds. With this newly gained olfactory intelligence, ugo robots will expand their real-world capabilities by being able to detect gas leaks, toxic chemicals, and equipment failures, monitor air quality and pollutants, and more.
🧬 Biotechnology
▶️ The Ultimate Guide To Genetic Modification (41:25)
In this video, The Thought Emporium, YouTube’s chief mad scientist, gives an excellent introduction to genetic engineering and DNA printing. Starting with simple fluorescent proteins and progressing to complex genetic logic gates and oscillators, the video explores how DNA functions as a biological programming language. Along the way, it covers lab techniques, synthetic biology concepts, and the launch of a new open-source plasmid store designed for students, hobbyists, and bio-nerds alike.
AstraZeneca pays up to $1bn for biotech firm ‘that could transform cell therapy’
AstraZeneca is acquiring Belgian biotech firm EsoBiotec for up to $1 billion ($425 million upfront, $575 million in milestones). EsoBiotec specialises in in-vivo CAR-T cell therapies, which genetically engineer immune cells directly inside the patient’s body. This method could transform cell therapy by making treatments faster, cheaper, and more accessible—administered in minutes instead of weeks, and potentially at a fraction of the cost (around $450,000–$500,000 for current therapies).
Researchers engineer bacteria to produce plastics
Scientists in Korea have engineered a strain of E. coli bacteria that can produce biodegradable plastic using only glucose as a fuel source. By tweaking natural enzymes and introducing genetic modifications, the team enabled the bacteria to synthesise flexible polymers made from amino acids and other small molecules, potentially offering an eco-friendly alternative to traditional plastics. The resulting material is biodegradable and can be tailored for different properties, though challenges remain in controlling polymer composition, scaling up production, and purifying the product.
AI-Designed Enzymes
Creating entirely new enzymes—proteins that perform specific chemical reactions—from scratch is one of the ultimate goals of protein design. A team of researchers from David Baker’s lab at the Institute for Protein Design has taken a step towards realising that goal by successfully creating complex enzymes—specifically serine hydrolases—from scratch using AI-driven tools. By combining machine learning models such as RFDiffusion, AlphaFold2, and a new filtering method called PLACER, the team boosted enzyme functionality from 1.6% to 18%, with two designs achieving full catalytic activity, meaning that the designed enzyme was able to complete the entire multi-step reaction cycle, just like a natural enzyme would. Though slower than natural enzymes, the work marks a significant step towards designing powerful, custom-made enzymes for medicine, industry, and beyond.
💡Tangents
AlexNet Source Code Is Now Open Source
AlexNet is one of the most important and impactful algorithms in the history of computing. Developed in 2012 by graduate students Alex Krizhevsky and Ilya Sutskever, along with their faculty advisor Geoffrey Hinton, AlexNet demonstrated the potential of running deep neural networks on GPUs. The algorithm decisively won the ImageNet challenge and kickstarted the AI revolution that continues to this day. Thanks to efforts by the Computer History Museum, the original AlexNet code has been recovered and made open source, and it is now available for everyone to view and study on GitHub.
Thanks for reading. If you enjoyed this post, please click the ❤️ button or share it.
Humanity Redefined sheds light on the bleeding edge of technology and how advancements in AI, robotics, and biotech can usher in abundance, expand humanity's horizons, and redefine what it means to be human.
A big thank you to my paid subscribers, to my Patrons: whmr, Florian, dux, Eric, Preppikoma and Andrew, and to everyone who supports my work on Ko-Fi. Thank you for the support!
My DMs are open to all subscribers. Feel free to drop me a message, share feedback, or just say "hi!"